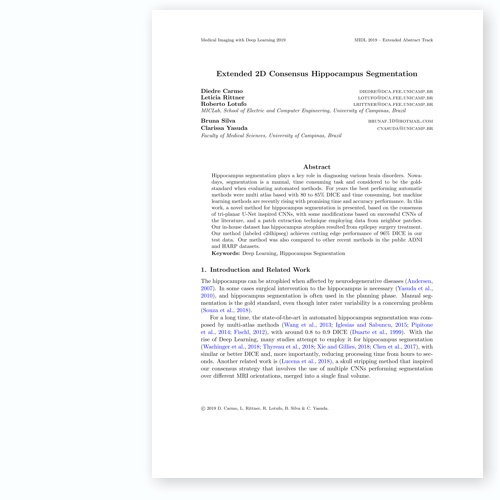
Extended 2D Consensus Hippocampus Segmentation
Medical Imaging with Deep Learning 2019
Hippocampus segmentation plays a key role in diagnosing various brain disorders. Nowadays, segmentation is a manual, time consuming task and considered to be the gold-standard when evaluating automated methods. For years the best performing automatic methods were multi atlas based with 80 to 85% DICE and time consuming, but machine learning methods are recently rising with promising time and accuracy performance. In thiswork, a novel method for hippocampus segmentation is presented, based on the consensus of tri-planar U-Net inspired CNNs, with some modifications based on successful CNNs of the literature, and a patch extraction technique employing data from neighbor patches. Our in-house dataset has hippocampus atrophies resulted from epilepsy surgery treatment. Our method (labeled e2dhipseg) achieves cutting edge performance of 96% DICE in ourtest data. Our method was also compared to other recent methods in the public ADNI and HARP datasets.
Full paper here: https://openreview.net/pdf?id=Sygx97DaKV
Publication Info
- Category: Algorithms | Brain | Pattern recognition | 
- Authors: Diedre Carmo, Letícia Rittner, Roberto Lotufo, Bruna Silva, Clarissa Yasuda
- How to cite: Carmo, D.; Rittner, L.; Lotufo, R.; Silva, B.; Yasuda, C. MIDL2019 – Extended Abstract Track: "Extended 2D Consensus Hippocampus Segmentation". Medical Imaging with Deep Learning, 2019.
- Published: 2019