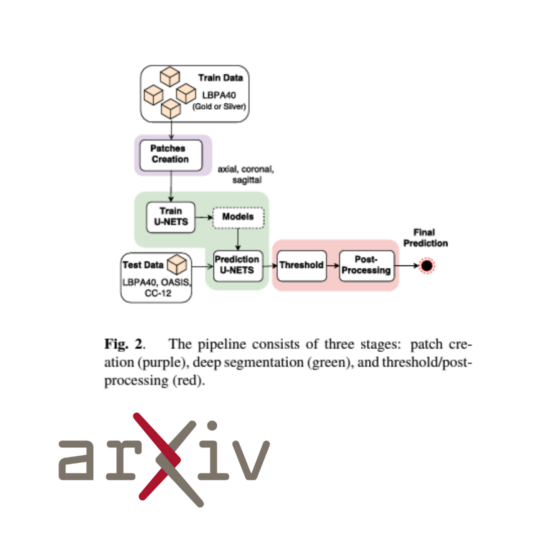
Silver Standard Masks for Data Augmentation Applied to Deep-Learning-Based Skull-Stripping
arXiv:1710.08354v1 [eess.IV] 23 Oct 2017
The bottleneck of convolutional neural networks (CNN) for medical imaging is the number of annotated data required for training. Manual segmentation is considered to be the “gold-standard”. However, medical imaging datasets with expert manual segmentation are scarce as this step is time-consuming and expensive. We propose in this work the use of what we refer to as silver standard masks for data augmentation in deep-learning-based skull-stripping also known as brain extraction. We generated the silver standard masks using the consensus algorithm Simultaneous Truth and Performance Level Estimation (STAPLE). We evaluated CNN models generated by the silver and gold standard masks. Then, we validated the silver standard masks for CNNs training in one dataset, and showed its generalization to two other datasets. Our results indicated that models generated with silver standard masks are comparable to models generated with gold standard masks and have better generalizability. Moreover, our results also indicate that silver standard masks could be used to augment the input dataset at training stage, reducing the need for manual segmentation at this step.
Full paper here: https://doi.org/10.48550/arXiv.1710.08354
Publication Info
- Category: Brain | 
- Authors: Oeslle Lucena, Roberto Souza, Letícia Rittner, Richard Frayne, Roberto Lotufo
- How to cite: LUCENA, Oeslle et al. Silver standard masks for data augmentation applied to deep-learning-based skull-stripping. In: 2018 IEEE 15th International Symposium on Biomedical Imaging (ISBI 2018). IEEE, 2018. p. 1114-1117.
- Published: 23 October 2017